Modality Compensation Network:
Cross-Modal Adaptation for Action Recognition
Abstract
With the prevalence of RGB-D cameras, multimodal video data have become more available for human action recognition. One main challenge for this task lies in how to effectively leverage their complementary information. In this work, we propose a Modality Compensation Network (MCN) to explore the relationships of different modalities, and boost the representations for human action recognition. We regard RGB/optical flow videos as source modalities, skeletons as auxiliary modality. Our goal is to extract more discriminative features from source modalities, with the help of auxiliary modality. Built on deep Convolutional Neural Networks (CNN) and Long Short Term Memory (LSTM) networks, our model bridges data from source and auxiliary modalities by a modality adaptation block to achieve adaptive representation learning, that the network learns to compensate for the loss of skeletons at test time and even at training time. We explore multiple adaptation schemes to narrow the distance between source and auxiliary modal distributions from different levels, according to the alignment of source and auxiliary data in training. In addition, skeletons are only required in the training phase. Our model is able to improve the recognition performance with source data when testing. Experimental results reveal that MCN outperforms state-of-the-art approaches on four widely-used action recognition benchmarks.
Framework
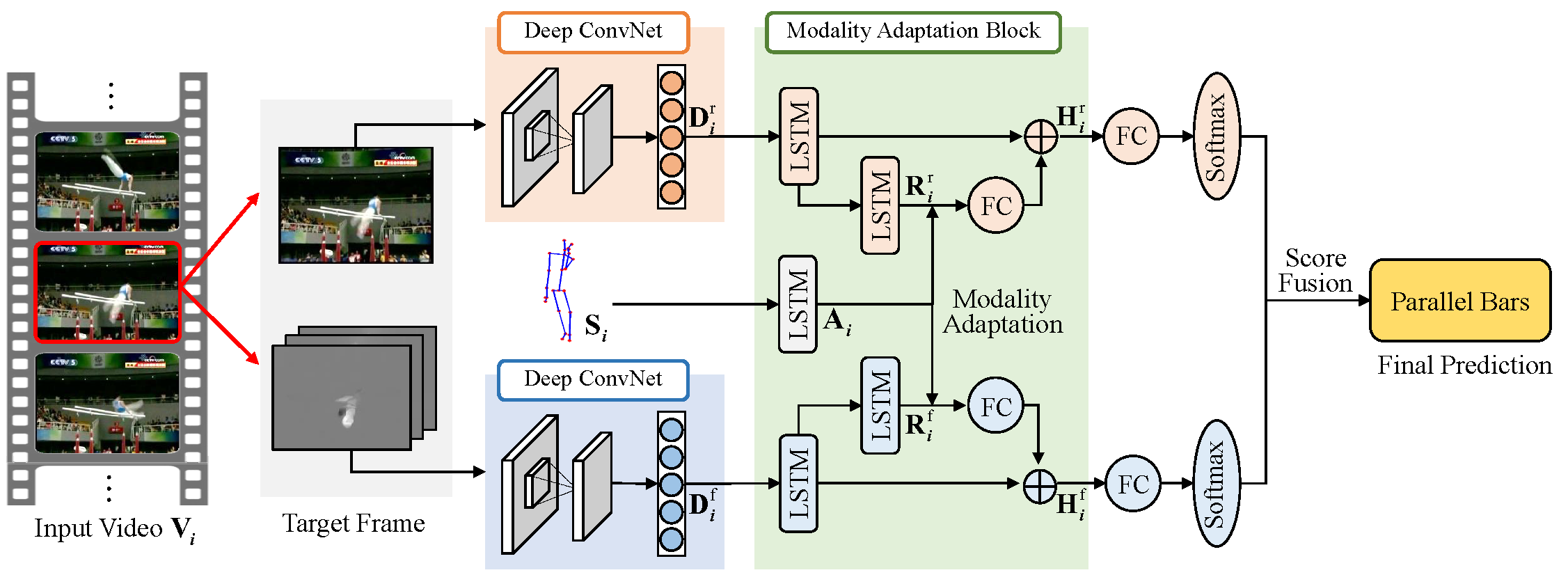
Fig.1 The framework of Modality Compensation Network. Based on the two-stream architecture, a modality adaptation block is incorporated to compensate the feature learning of source data with the help of auxiliary data. (We use superscripts 'r' and 'f' to indicate RGB and flow streams, respectively. Note that the LSTM with output $A_i$ is not required in the testing phase)
To deal with different source and auxiliary data sources, we consider different levels of modality adaptation, including domain-, category-, and sample-level adaptation:
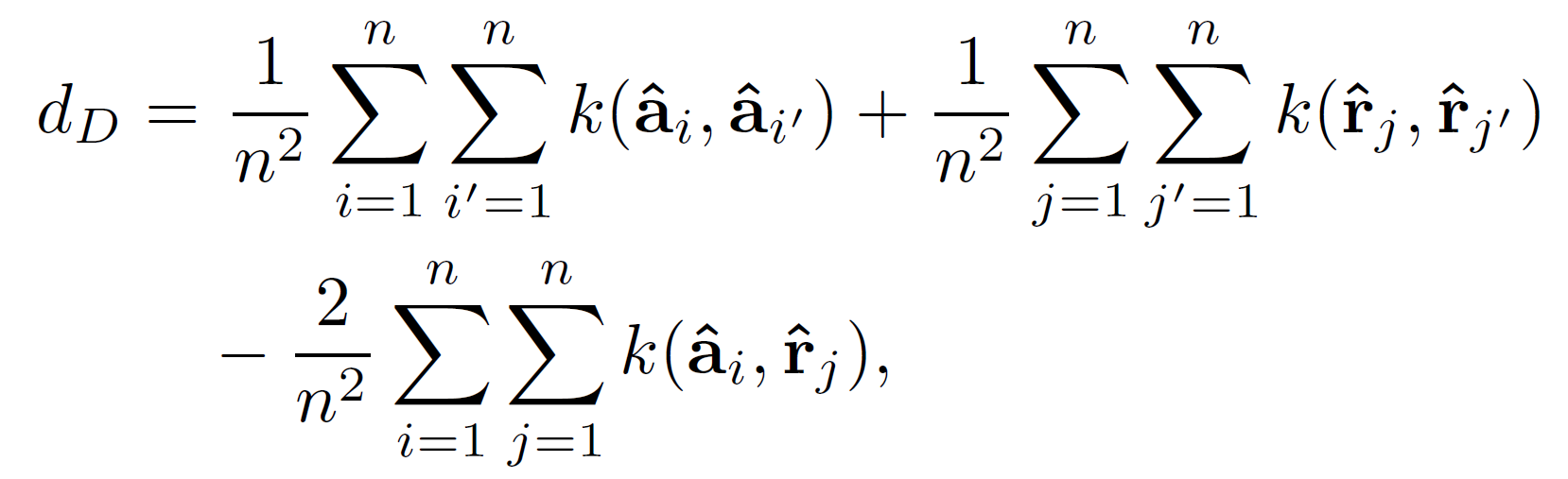
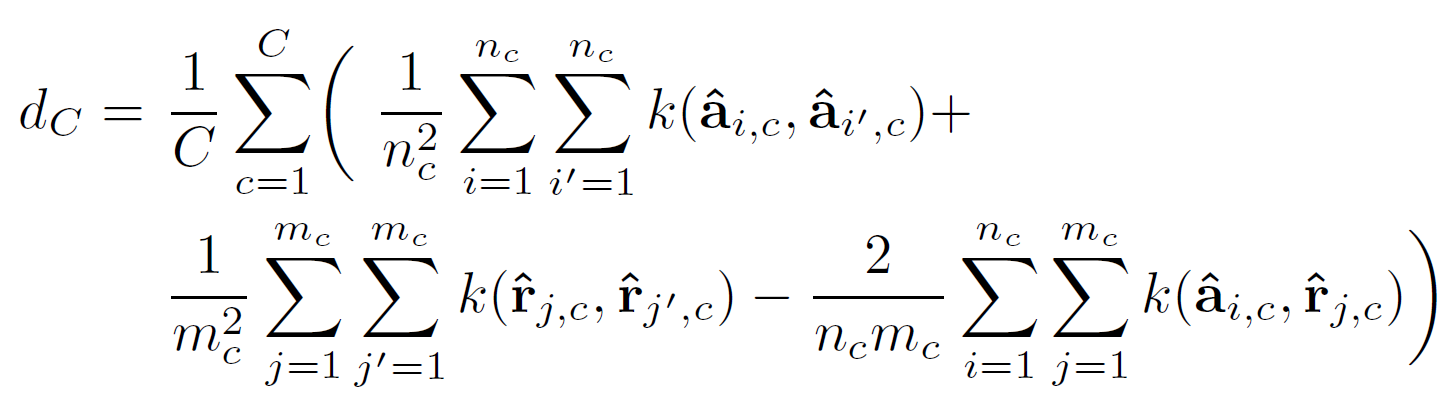
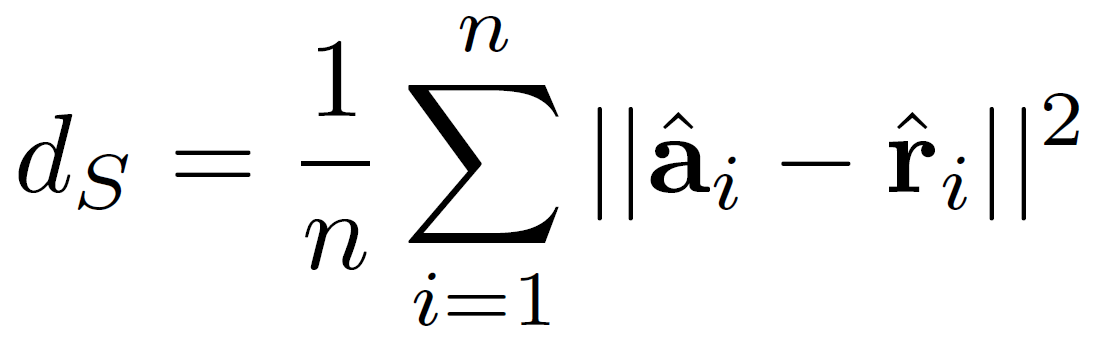
Results
We conduct experiments with the configurations targeting different cases as follows:
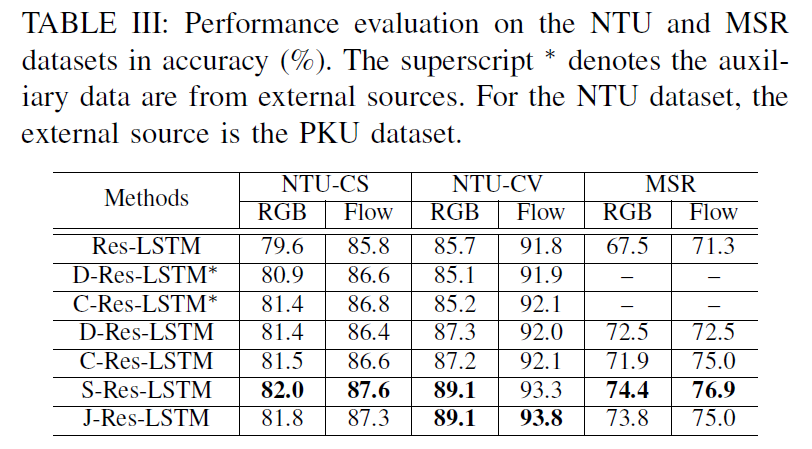
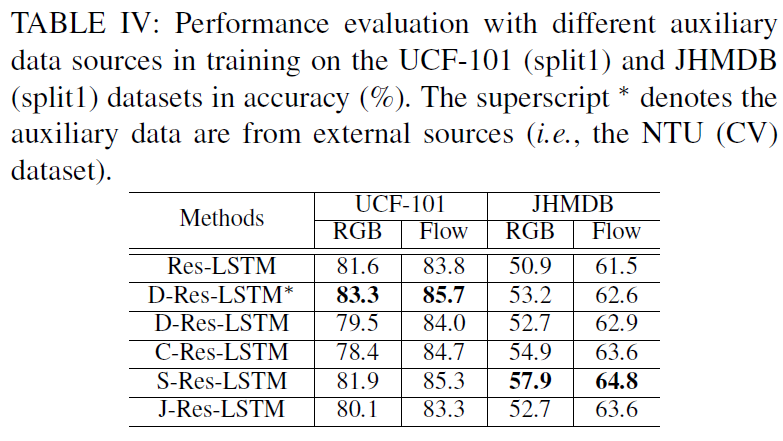
For more results compared with state-of-the-art methods, please refer to our paper.
Resources
Citation
@article{song2020Modality, title={Modality Compensation Network: Cross-Modal Adaptation for Action Recognition}, author={Song, Sijie and Liu, Jiaying and Li, Yanghao and Guo, Zongming}, journal ={IEEE Transactions on Image Processing}, year={2020} }
Reference
[1]. A. Gretton, K. M. Borgwardt, M. J. Rasch, B. Sch¨olkopf, and A. Smola, “A kernel two-sample test,” Journal of Machine Learning Research, vol. 13, pp. 723–773, 2012.